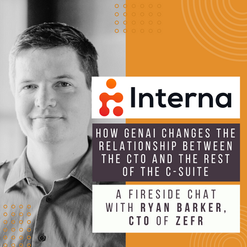
A Fireside Chat with Ryan Barker, CTO of Zefr
In this fireside chat, I talk with Ryan Barker, the CTO of Zefr. Ryan faces interesting challenges. His company is on the leading edge of...
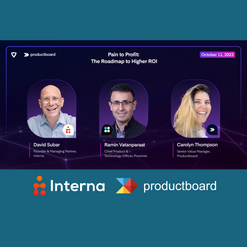
Product Excellence Summit - Pain to Profit
In October I spoke on a panel at the Product Excellence Summit put on by #ProductBoard. We talked about feature factories, and how to...

Interna Talks 4 - OpenAI, Vendor Dependence, and What has Changed About Product Management
Welcome, everyone, to another set of Interna talks, where I get to talk with my colleagues here at Interna. We talk about some of the...